Hannah Sutton, New Science Communicator
These days, people think that most of our questions about the world can be answered with science. However, it’s not always possible to study something directly, which is where mathematical modelling comes in.
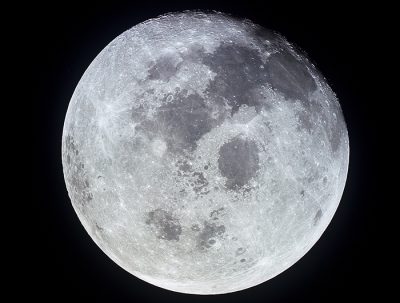
The moon photographed from Apollo 11 spacecraft. Photo: NASA AS11-44-6667; public use
For example, for quite a while scientists were baffled by the fact that the near side of the moon appeared to have fewer craters than the far side of the moon. Many scientists believed thatthe Earth shielded the near side of the moon from incoming asteroids, thus the un-shielded, far side received more impacts. However, by using simple straight-line geometry, researchers calculated that the Earth itself is not a big enough shield to account for the lack of craters on the far side of the moon.
It is impossible to go back in time and observe the formation of moon craters in person, and ethical reasons prevent us from launching missiles at the moon in order to physically re-create craters.
Therefore, by utilizing data such as a revised measurement of the size distribution of lunar craters, and information on the local magnetic fields on the moon, a mathematical model was developed by Laurence A. Soderblom for small-impact erosion applied to the lunar surface. Using Soderblom’s model, scientists were able to simulate that the moon previously had approximately the same number of craters on both sides but, due to the thinner crust on the near side, volcanoes were able to erupt and fill in ancient large craters. Thus those craters from the moon’s early history were no longer visible, leading to the observation of fewer craters on the near side of the moon.
This is an example of how scientists use mathematical models when it’s impossible to use direct measurements. Mathematical modelling works on the hope that one can solve any problem mathematically; however, this is quite ambitious and, if it were true, then mathematicians would rule the world.
Therefore we re-define mathematical modelling as the assumption that one can attempt to approximateanything. Mathematical modelling contributes to scientific discovery because there are numerous open problems in science where data cannot be collected or measured through current engineering or scientific methods. It is also valuable to compare mathematical models to measured data to test model accuracy.
One of the problems that mathematical modelling can help solve is the temperature regulation of a stationary swarm of bees. Recent research about honeybee swarms, conducted by former Simon Fraser University student Jeremy Chiu under the supervision of math professors J.F. Williams and John Stockie, used modelling to study honeybee swarms.
Swarming is when a cluster of honeybees leave their current hive to establish a new colony somewhere else. Once out of the hive, the majority of the bees remain stationary on a tree branch whilst other scouts look for a new home. A well-documented but not well understood phenomena of honeybees is how they regulate temperature in a stationary swarm. The swarm may remain on the branch for several days, leaving it exposed to the elements, but the bees are able to regulate cluster temperature at comfortable levels.
The swarm does not necessarily maintain the same temperature on the outside as in the inside. For example, swarms of 1,000 to 3,000 bees can maintain their core swarm temperature to within one degree of 35°C, while keeping the exterior, or outermost layer of bees, at a temperature between 19°C and 35°C.
It is difficult to directly measure swarm temperature for scientific analysis. Factors such as environment – that is, whether the bees are in nature or in a lab – could affect the data. It is also difficult to find a swarm of honeybees to measure. Also, bees are commonly euthanized after experiments so that they can be counted, but this is a questionable approach given their dwindling populations.
Luckily, this phenomenon lends itself nicely to mathematical modelling, as the bees seem to follow a predictable pattern by moving within the swarm to adjust their desired temperature or density.

Photo by Florida Division of Plant Industry, Florida Department of Agriculture and Consumer Services, CC BY 3.0 – Bugwood.org
Chiu’s model examines how bees move to adapt to change in temperature within the swarm, using two dependent variables: temperature, Ï„, and the density of bees, Ï. To model the temperature within the swarm, he uses a partial differential heat equation, which “has a diffusive term that captures the mechanism of heat conduction between the bee’s bodies, and a source term that describes heat generated by bees shivering.” As for density, “bees don’t want to be too hot, cold, lonely, or crowded. So we assume that, when bees are cold, they move up the temperature gradient, in the direction where they’ll warm the fastest,” says Chiu. Another parameter considered in Chiu’s is the radius of the swarm.
Chiu verifies the accuracy of his model by comparing the model output to data measured by scientists, specifically work conducted by Bernd Heinrich. In Chiu’s numerical simulation, “the maximum and minimum temperatures were 33.6°C and 17.3°C, respectively, which is quite close to Heinrich’s measured 35°C and 19°C.” However, Chiu didn’t have a measured density profile against which to compare his simulated profile.
Using this model, biologists can measure differences in bee swarm behavior, whether there are 1,000 or 5,000 bees. If Chiu’s model is sufficiently accurate, biologists could run hundreds of simulations by computer if they wanted to test model accuracy, or simply change the value of each parameter to observe different outcomes. Thus field studies that would usually take weeks to complete and generate research costs could be done over a shorter time period for minimal cost.
Naturally, we must never assume that mathematical models are perfect representations of reality. Although the statistical accuracy of simulations can be high due to the set-up of the model and the variables and parameters used, with any simulation we need to question whether the model accurately represents a biological phenomenon. However, when it is hard or impossible to measure real data, mathematical models are ideal to investigate scientists’ hypotheses.
~30~